GEEK HUB
Optimization and robust pizza camera design
By Wendy Luiten
In 1965 Intel's George Moore predicted that available computing power would effectively double every year. "Moore's Law" has held up empirically for more than 55 years. That increase in computing power has allowed engineering simulation to become an essential part of the product design process. But it is much less known that the advances in computer power and computational tool development can be used to guide a design, and to develop the best possible design solution in a structured way, using data driven decisions based on the results of multiple computer simulations.
A news item inspired the following fairy tale on the thermal design of a Pizza Camera, illustrating the possibilities. The case itself is fictional, but the content is based on the authors practice as a thermal expert and a certified Master Black Belt in Innovation Design for Six Sigma. (DfSS).
Requirement elicitation: The story starts with the business idea for a pizza surveillance camera for the pizza food industry. Stakeholders are approached for requirements: The pizza cook says it needs to work in a hot kitchen and show if the toppings are put on correctly. The food safety specialist wants it to measure temperatures accurately, be easy to clean, and be reliable. And the device installers want it to be small and compatible with the accessory line. In the end, the requirements state a small size of about 5 x 5 x 5 cm, in a closed box, containing a color and IR image sensor and video processor, with a max ambient of 50ºC and a wish for 65ºC, and high reliability and accuracy – starting from 95%.
First concept
The first concept features a sealed enclosure of 50 x 50 x 60 mm, containing 3 critical components with a max device temperature of 80ºC. Total heat dissipation is 6.6 W. A CFD simulation on this first concept shows that this design is not feasible thermally.
Digital design optimization
After a fruitful brainstorm, the development team comes up with alternatives for 5 design decisions. But how to find out what alternatives are best? Design of Experiments (DoE) is a technique that compares the results of groups of experiments to separate effects. Crucially, multiple inputs are changed at the same time, as this enables to find interactions, cases where design choices strengthen each other. In this case, a 16 run partial factorial DOE design was run with 2 options for each of the 5 decisions. Using the parametric capabilities of the computational tool, this is as easy as setting up the DOE matrix, let this solve automatically, process the results, choose the best design and verify with a computer simulation incorporating all design choices.
The best concept is already 64ºC better … but it is still too hot. The team decides to use a smart temperature control to derate the device at high ambient. But how does the heat dissipation relate to the critical temperatures? A second DoE scenario is used, running variations with different component dissipations, different ambient temperatures and whether there is an external housing yes or no. Analysis yields the relation between the critical component temperature, ambient and heat dissipation. Assuming a duty cycle type control with 100% performance corresponding to the nominal 6.6 W heat dissipation, a derating of 10% heat would correspond to 90% heat dissipation and 90% performance. It turns out that for ambient temperatures below 50ºC no derating is necessary. Without external housing, derating starts at 51ºC and at 65ºC performance (duty cycle) is at 37% . With housing, derating starts at 59ºC and performance at 65ºC ambient is at 73% ambient.
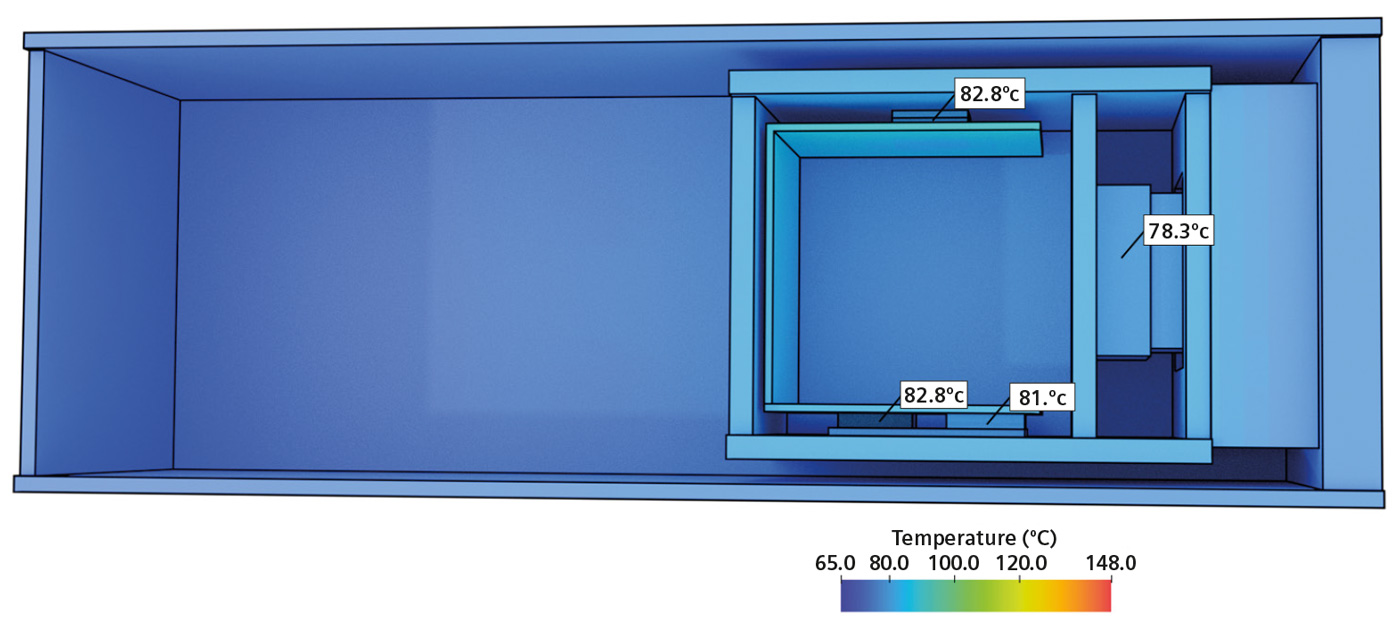
Robust design
Manufacturing spread and differences in application software cause a +/- 25% uncertainty in the heat dissipation. This can be lowered to +/- 10% when a low-power mode is activated when derating. In addition, the tempearature sensor has a measurement error of +/- 6ºC before and 0.6ºC after calibration. The variations are assumed to have a normal (gaussian) distribution with a range of +/- 3 standard deviations. Recalculating the performance many times with slightly different heat dissipations and temperature measurement errors results in the expected distribution of the performance over many cameras. This procedure is called Monte Carlo Simulation. In case of the pizza camera, we assume that the variations in heat and sensor are normally distributed with a range of +/- 3 standard deviations. The new calculations show that the performance becomes a wide band instead of a curve. When the variability in heat dissipation and sensor error is reduced, the bands become narrower, and the behavior is more predictable.
To gain insight into how this unpredictability will affect the customer experience, we look at the distribution at a fixed temperature. At 65ºC ambient, the camera with external housing has an expected performance of 73%. Let’s assume that a customer is satisfied if the actual performance is between 68% and 78%. A second Monte Carlo simulation demonstrates that without mitigation, only 36% of pizza cameras will meet expectations. About 1/3rd derates too much resulting in unnecessary slow applications and blocky images and about 1/3rd derates too little, resulting in pictures with image noise. With reduced variability 95% meets the expected performance.
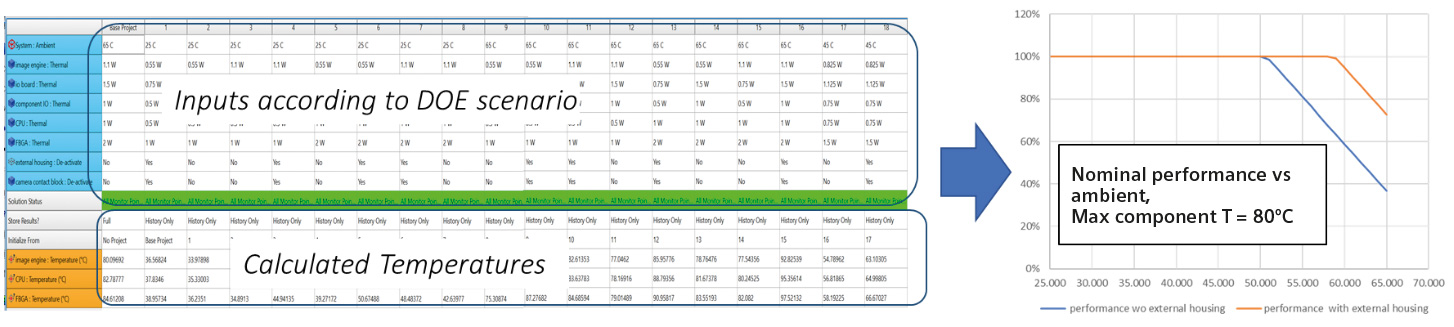
In conclusion
The pizza camera story is a purely fictional example, based on news items on a pizza camera and combining thermal design and statistical cases from the author’s practice. It serves the purpose of illustrating the power of digital design, using computer simulations not only as an after-the-fact design validation, but frontloading to enable data driven decisions during the design process. In this example, a total of 8 key decisions were made based on 2 DoEs with 16 runs each and 2 Monte Carlo simulations with 5000 runs, a modest effort in the digital domain but an unthinkable effort in hardware. In the end, the design is not only 62ºC cooler than the original, but there is also evidence that about 95% of the pizza cameras will perform as expected. The development team can enter the stage of hardware validation with confidence.
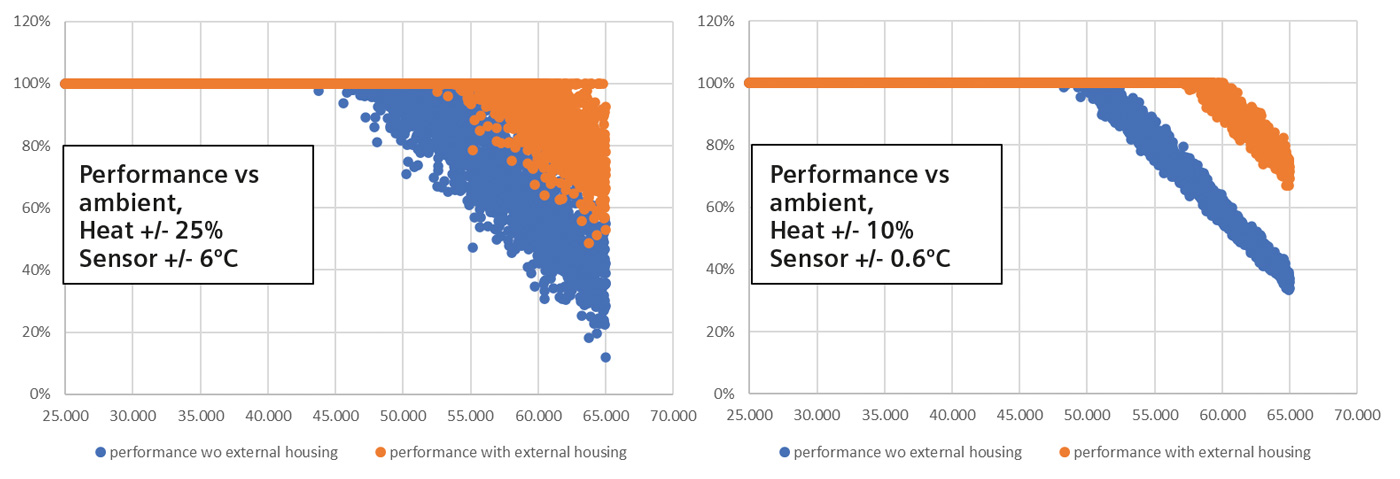
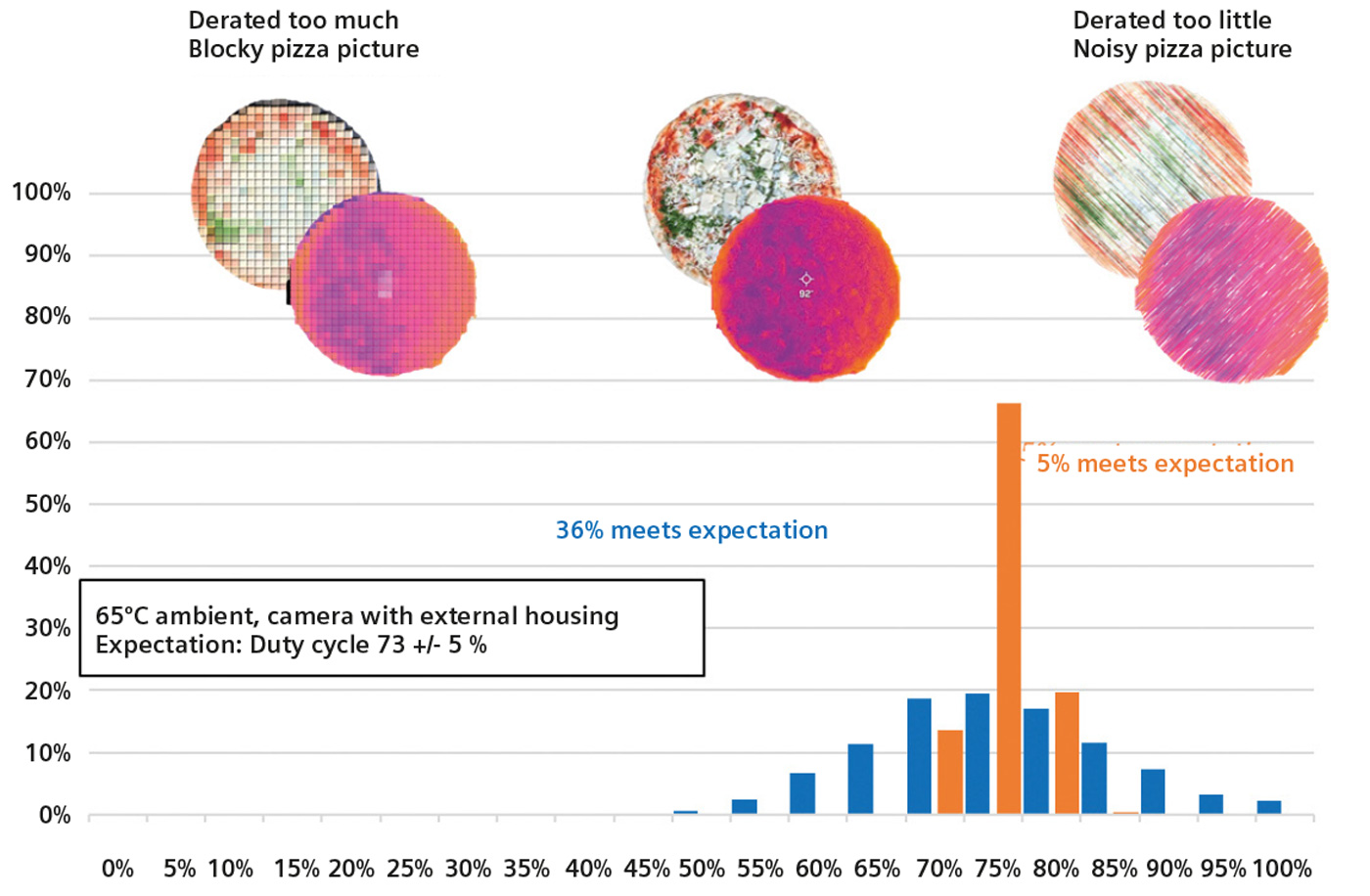